Revolutionizing Mental Health Treatment with Machine Learning and Neuroimaging Data
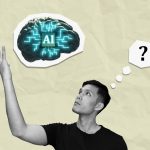
Antidepressants are a widely used treatment for various mental health conditions, including depression and anxiety. Despite their prevalence, challenges persist in understanding who will benefit from antidepressant treatment. The majority of people with Major Depressive Disorder (MDD) do not achieve remission after initial antidepressant therapy, raising concerns about side effects and medication withdrawal when used long-term.
As the world grapples with the mental health crisis exacerbated by the COVID-19 pandemic, there is a pressing need for innovative approaches. Data science and machine learning offer promising avenues for advancement in mental health treatment, with the emergence of ‘data-driven psychiatry’ on the horizon.
The Power of Machine Learning in Predicting Treatment Response
Machine learning (ML) involves computers learning from data to make decisions or predictions without explicit programming. ML models can uncover intricate relationships between variables and outcomes without predefined hypotheses, distinguishing them from traditional statistical methods. In a recent study, researchers evaluated ML models to predict patient response to the antidepressant sertraline using data from a randomized controlled trial (RCT).
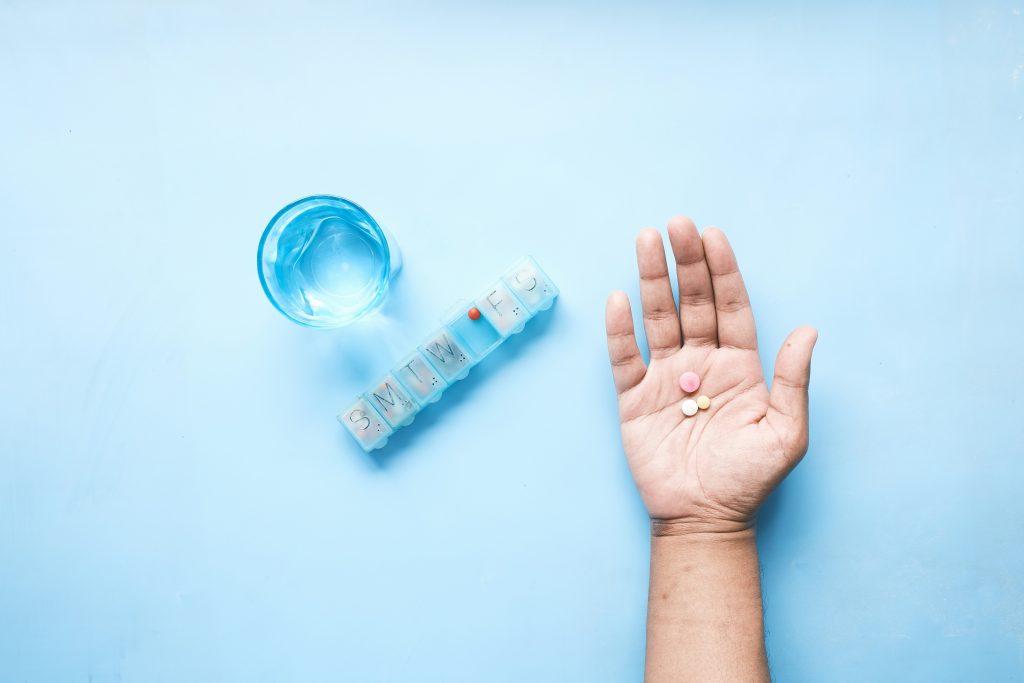
Research suggests that about two-thirds of antidepressant users don’t respond to initial treatment. Machine learning models may help clinicians identify those patients likely to be at risk at an early stage.
Understanding the Methods and Results
The study utilized the XGBoost ML algorithm to predict patient response to sertraline in early treatment stages. By training the model on data from the EMBARC clinical trial, the researchers identified clinical and neuroimaging data as key predictors of treatment response. The analysis revealed a balanced accuracy of 68% in predicting sertraline response at week 8 from measurements taken in the early treatment phase.
Models trained on robust predictors such as age, hippocampal volume, and symptom reduction demonstrated superior performance compared to models relying on weaker predictors. Interestingly, arterial spin labelling (ASL) features, a neuroimaging technique measuring cerebral blood flow, emerged as a significant pre-treatment predictor. Clinical markers, including changes in depression scores, were crucial for predicting early treatment response.
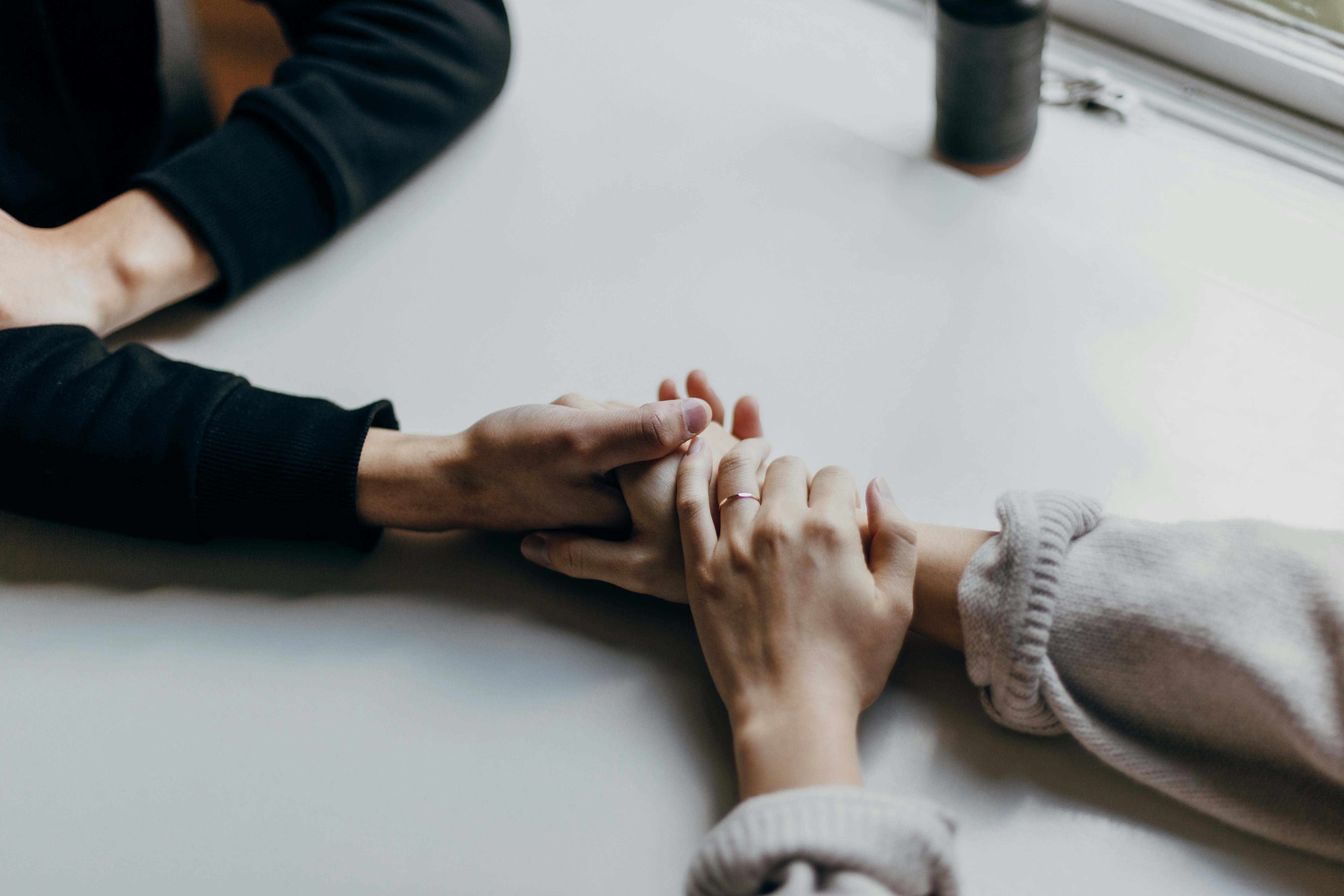
Machine learning methods may predict sertraline response at week 8 from early treatment measurements at week 1.
Implications and Limitations
The study’s findings spotlight the potential of integrating clinical and neuroimaging data for enhanced antidepressant response prediction. While neuroimaging data showed promise, the superior performance of clinical data raises questions about the practicality and cost-effectiveness of routine neuroimaging assessments in psychiatric care.
Further exploration into the predictive power of ASL perfusion data and the balance between clinical and neuroimaging predictors is warranted. The comparison with traditional treatment prediction methods underscores the need for continued research into optimizing treatment planning for individuals with depression.
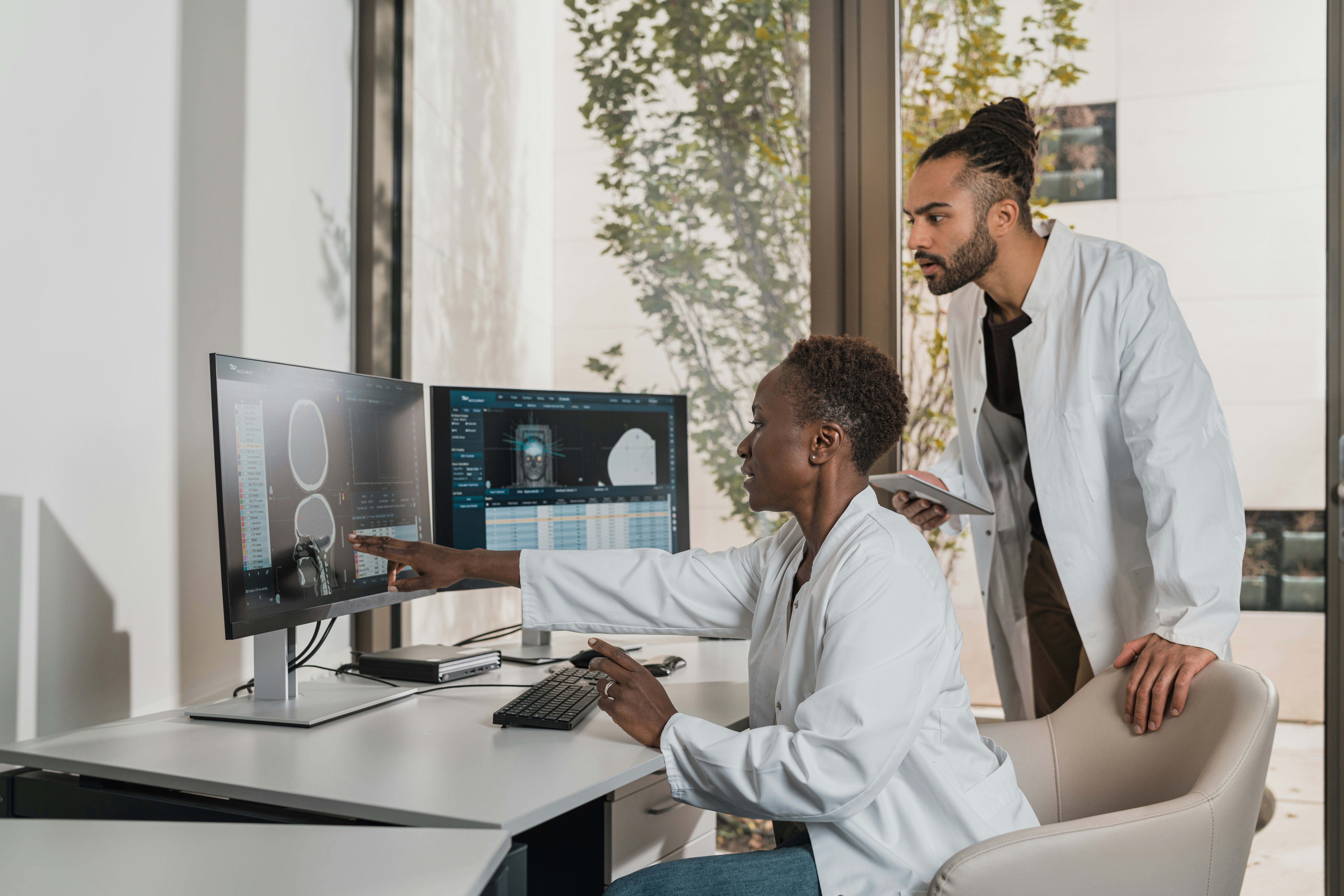
Clinical and neuroimaging data both play critical roles in predicting antidepressant response in depression patients.
Real-World Applications and Future Directions
While the study sheds light on the potential benefits of utilizing ML models for treatment planning, considerations about the practical implementation of neuroimaging data in clinical settings remain paramount. The balance between predictive accuracy and cost implications necessitates a holistic evaluation of the value proposition offered by advanced prediction models.
As we navigate the evolving landscape of mental health treatment, leveraging data-driven insights to tailor interventions to individual needs holds promise. The intersection of data science, neuroimaging technology, and clinical practice presents an exciting frontier in mental health care, paving the way for personalized and effective treatment strategies.
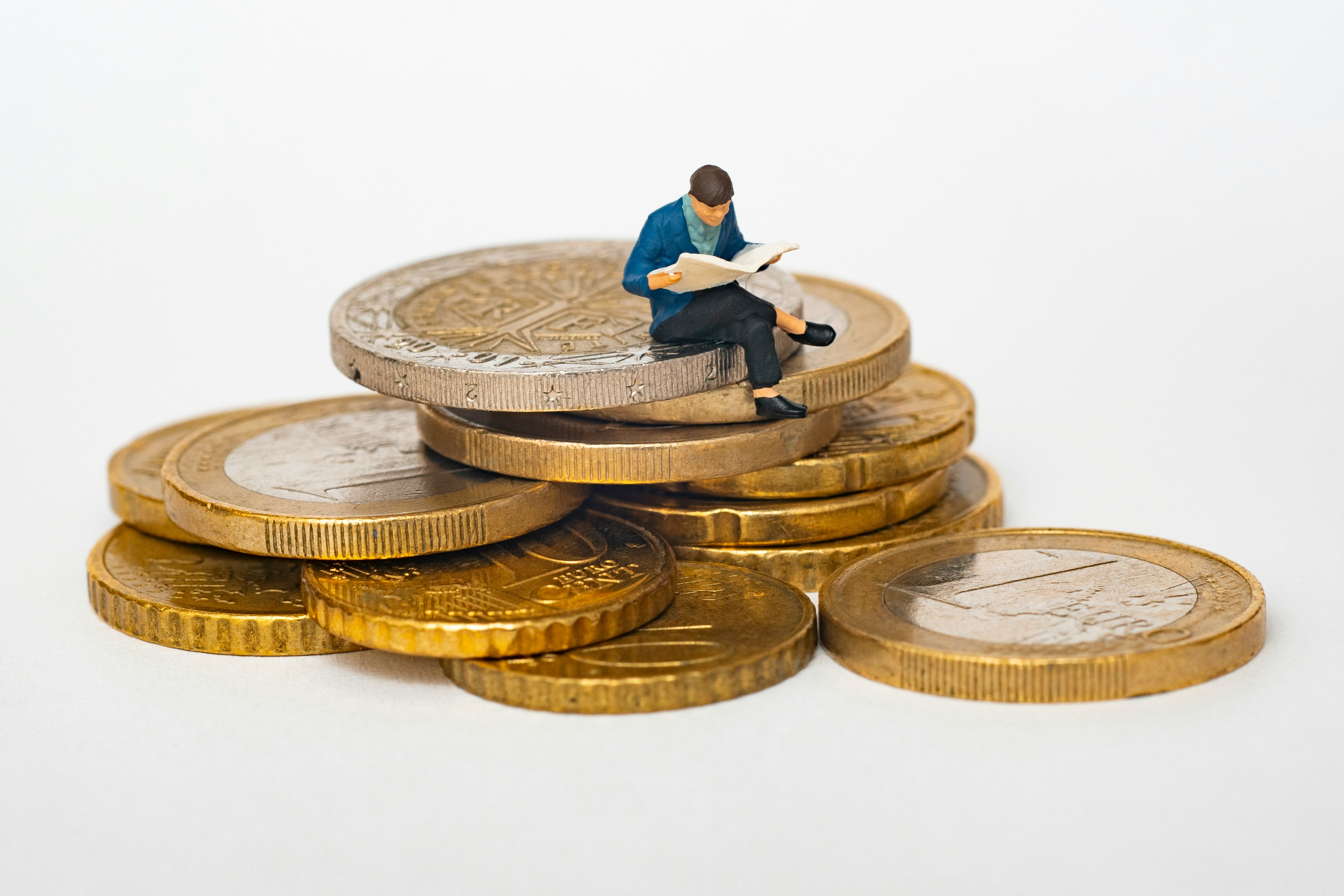
Optimizing mental health treatment through predictive models offers potential cost savings and improved patient outcomes.
Conclusion
The convergence of machine learning, neuroimaging, and clinical data heralds a new era of precision psychiatry. By harnessing the power of predictive models, clinicians can tailor treatment plans to individual needs, enhancing outcomes and reducing the burden of trial-and-error approaches to medication management.
The journey towards data-driven mental health care is filled with promise and challenges, requiring a collaborative effort across disciplines to unlock the full potential of advanced technologies in improving patient care and well-being.
Statement of Interests
No conflicts of interest to declare.
References
Primary Paper
Maarten G Poirot, Henricus G Ruhe, Henk-Jan M M Mutsaerts, Ivan I Maximov, Inge R Groote, Atle Bjørnerud, Henk A Marquering, Liesbeth Reneman, Matthan W A Caan. (2024) Treatment Response Prediction in Major Depressive Disorder Using Multimodal MRI and Clinical Data: Secondary Analysis of a Randomized Clinical Trial. Am J Psychiatry. Am J Psychiatry 181, 223-233 (2024). https://doi.org/10.1176/appi.ajp.20230206
Other References
Medicines Used in Mental Health – England – 2015/16 to 2022/23; NHSBSA (2023).
Keks, N., Hope, J. & Keogh, S. Switching and stopping antidepressants. Aust Prescr 39, 76–83 (2016).
Ionescu, D. F., Rosenbaum, J. F. & Alpert, J. E. Pharmacological approaches to the challenge of treatment-resistant depression. Dialogues Clin Neurosci 17, 111–126 (2015).
Coronavirus and depression in adults, Great Britain: July to August 2021; Office for National Statistics (2021).
Mental health crisis care services ‘under-resourced, understaffed and overstretched’, Mind.
What is Machine Learning? Definition, Types, Tools & More, datacamp (2023).
Trivedi, M. H. et al. Establishing moderators and biosignatures of antidepressant response in clinical care (EMBARC): Rationale and design. J Psychiatr Res 78, 11–23 (2016).
Clement, P. et al. A beginner’s guide to arterial spin labeling (ASL) image processing. Sec. Neuroradiology 2, 1-12 (2022).